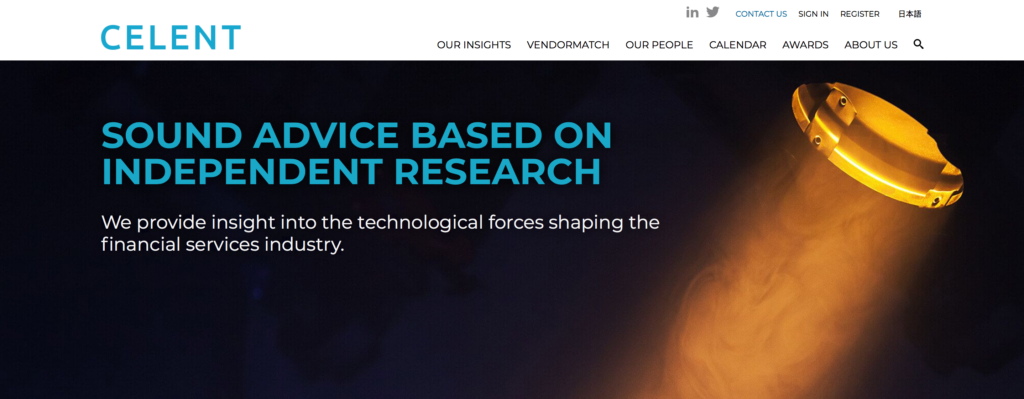
From banking chatbots to speculations on superintelligence, the impact of artificial intelligence (AI) on financial services is one of the hottest topics in fintech. Our Summit Day sessions on AI at FinovateSpring earlier this year were consistently among our best attended sessions.
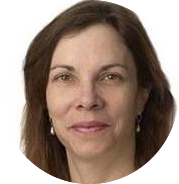
To continue this conversation, we exchanged emails with Alenka Grealish, Senior Analyst, Corporate Banking, Celent. Grealish’s recent report, AI in the UI: Adoption, Use Cases, and Business Cases, represents Celent’s latest investigation into the issues surrounding the rise and role of AI in financial services.
Finovate: In setting up this conversation, I noted that Celent referred to this as part of an inaugural initiative. Why is now the time to turn the spotlight on this technology and its impact on financial services?
Alenka Grealish: We observed the beginning of a shift from all experimentation to gradual implementation amongst vanguard banks. It was common to have nine proofs of concept to one pilot at the vanguard banks. We’re now seeing more pilots and a few moving into production.
Finovate: What are we talking about when we talk about AI? How broad is this technology?
Grealish: Broad. What defines AI has expanded in the commercial world. The narrow Turing Test no longer applies. The current goal of AI developers is not to replicate humans, but rather complement them and build applications that team with them. A great example is found in anti-money laundering.
The broad definition includes rules-based and learning-based models. A useful way to categorize AI capabilities is: natural language processing and understanding, natural language generation (data-to-text), speech (speech-to-text and vice versa), vision, and data insights (machine learning driven analytics that generate, for example, cash flow forecasts for customers and next best action for bankers).
Finovate: Has AI become a catch-all for a variety of technologies, some of which are AI and some of which are not? And is that an issue for AI adoption going forward?
Grealish: AI has certainly become a buzz word and its definition stretched by tech vendors. Semantics aside…Critical to successful AI adoption is not to seek a problem for AI to solve but rather the reverse: determine the key problems you’re trying to solve (e.g., high false positives in AML) and/or goals you’re trying to achieve (e.g., personalize customer-banker interactions). Then, (the next step is to) examine the potential means to solve/achieve. The means could be a combination of rules-based and learning-based AI or established tech (e.g., OCR) combined with AI.
Finovate: What were the top two or three high-level takeaways from your research?
Grealish: I was struck by the percentage of banks $10+ billion in size which had implemented front-office AI. I had expected less than 10%.
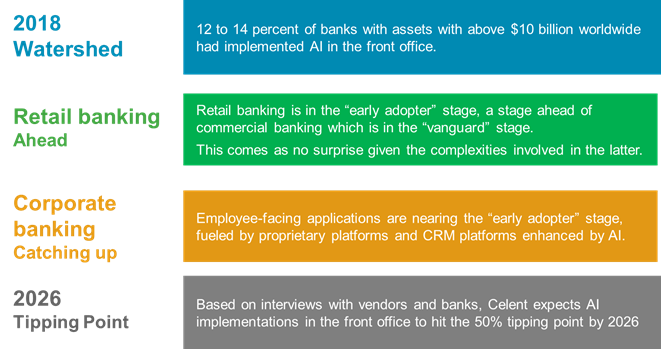
Finovate: Your report notes a difference in AI adoption between retail and commercial banks, calling the former an “early adopter” and the latter “vanguard.” What distinguishes the two?
Grealish: The vanguard phase is when a small number of entities, less than 5%, moves into production. The technology is not mature but works sufficiently well for low risk use cases. These entities tend to have nimble organizations and little to no legacy baggage. The early adopter phase typically occurs when the vanguard banks are successful and appear to be gaining a competitive edge and inspire the next wave of adopters to take action. The early adopters are innovators but are likely juggling multiple priorities and hence cannot always be in the vanguard.
Finovate: One of the areas you highlight is the use of AI-enabled technologies for employees and workers. What sort of use cases – especially those relevant to financial services – are you seeing here?
Grealish: In terms of employee enablement, I’m excited by what I see. AI is proving helpful in basic “tell me” support, such as, “do we offer this type of product?” and “where is this feature located in our online portal?” It is also progressing in higher level support, such as data insights on sales trends and next best action suggestions.
Finovate: You note “relative complexity” as a main hurdle to broader adoption of AI. How are financial services companies navigating this challenge (hiring talent, partnerships, etc.)?
Grealish: AI is not a standalone technology but rather is woven into current processes and platforms and/or drives new processes and platforms. Hence, success begins at the top of the house, banks with a transformation, data-driven leadership team view AI as one component of a broader digital strategy.
Next, successful banks have a business model comprising four key elements: a collaborative multidisciplinary organizational dynamic, an enterprise-wide AI initiatives team, strong data and model governance, and regulatory engagement and compliance playbook. At the operating model level, these banks have basic automation expertise and are incorporating AI to solve the hard stuff, such as analysis of unstructured data.
At the foundation, these banks are migrating to a modern data and tech infrastructure that supports a digital-first strategy.
Finovate: You note that the primary business goal for most businesses using AI-enabled technologies is cost savings, but that customer engagement “is increasingly a goal.” What are some of the more interesting use cases for AI-enabled technologies in customer engagement?
Grealish: We’re in the very early days of customer engagement, that is, brief, basic “tell me” conversations. These “tell me” conversations are taking off thanks to Siri, Alexa, Google Assistant, which are driving consumers’ comfort level engaging with machines. The outlook over the next 5 years is promising. “Do it for me” type interactions will become common. For example, a small business will simply ask the online virtual assistant to choose the optimal payment type based on its criteria. Further on the horizon are “Alert and advise me” type interactions. For example, a mid-market company has an FX exposure and is alerted with action options to hedge the exposure.